One of the things that many statistics students struggle with is on the quest to find the right analysis. While this should be praised, the hard reality is that in many situations, there isn’t one right analysis.
If you take a look at a textbook, for example, you will see that they only tend to show ideal situations and the right analysis. They can even be seen as cookbooks. You just need to follow the steps and to get the perfect meal. However, real data analysis is completely different. In fact, it rarely fits that ideal. And this is what makes data analysis so tough.
Discover everything you need to know about the z score table.
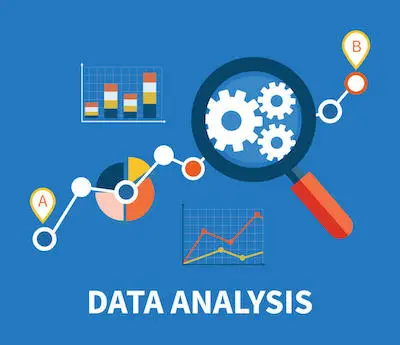
The truth is that with real data analysis, you need to keep in mind many different contextual factors. After all, you have your research question, the types of variables, the design, and even the data issues that need to come all together to do the best analysis you have available to you.
While you may have more control over what you have to work with when you are involved in the planning and execution of the data collection, your control also has a limit. And of course, sometimes you do everything right and things don’t go as you expected.
It’s not wrong to run an imperfect analysis as long as you’re transparent about its weaknesses. It doesn’t mean there is a better analysis out there.
Your job is to do the best analysis you can based on what you have to work with.
Looking to determine the Z value?
A Simple Example
Let’s take a look at a quick example so that you can fully understand what we mean here. Imagine that you have a dependent variable in the form of a rate. Imagine that it was the number of sales per employee. It was highly skewed. The unit of analysis was the sales office.
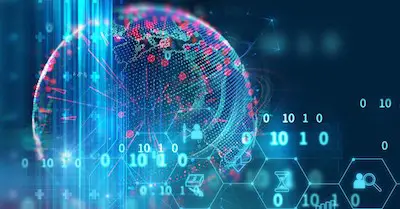
The best way to analyze these is with a count model – usually a Poisson or a negative binomial regression. Situations like this fit their assumptions. In case you don’t know, count models assume a skewed distribution of Y|X and the higher variance at higher means. In addition, they can include the number of employees as an exposure variable and will only give positive predicted values.
Make sure to use our z score calculator.
The problem is that count models require the dependent variable to be a count (number of sales). So, we have to separate the exposure variable (number of employees). The model will combine them into a rate.
But whoever collected these data combined these into a rate already. They didn’t keep the original variables. So the ideal analysis was just out of reach.
So, what could you do in this situation?
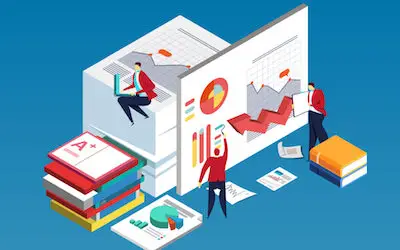
In our opinion, the best option would be a log transformation and a linear model instead. While it’s not ideal, this approach should mitigate issues with skew and non-constant variance. It can give a reasonable answer to the research question.
It’s important that the researcher describes in detail what he did and the possible biases and assumption violations this analysis introduces so that the reader can make their own inferences.